The automation of customer service through artificial intelligence has progressed remarkably, with chatbots handling a growing share of routine tasks. However, is AI truly ready to handle angry, frustrated customers?
While AI-powered chatbots using natural language processing (NLP) can now understand and respond to common customer questions and assist with basic troubleshooting, their ability to comprehend more nuanced conversations remains limited. In particular, current NLP systems face challenges in detecting frustrated tones and effectively handling complaints.
According to a study, 86% of customers still prefer to interact with a human agent when dealing with a complaint or complex issue. And irritated customers often have complex emotions, unique concerns, and specific needs that may extend beyond the capabilities of AI-driven systems. While an AI bot can offer trained responses and attempt to de-escalate situations, it may struggle to provide the level of empathy and understanding that human agents can.
AI holds the promise for transforming customer service, yet more development is still needed before it can reliably handle complex emotional exchanges. This article explores the progress and challenges of AI in customer service, analyzing when a human touch remains essential for resolving complaints and delivering genuine satisfaction.
Teaching Nuance: How AI Learns to Handle Customer Frustration
AI-powered chatbots efficiently handle high volumes of repetitive inquiries. However, when it comes to understanding the emotions of an angry customer or quickly assessing the need to hand off to a human agent, their capability can falter.
The goal of chatbot training for efficient and automated customer service is to instill human-like conversational skills to efficiently resolve complaints or discern when a human touch is required.
Chatbots leverage sophisticated machine learning and natural language processing (NLP) to comprehend and respond to customers. Through training, chatbots continuously learn from human-to-human service conversations to improve their language and dialog skills.
Training chatbots to proficiently handle frustrated customers involves more advanced methods focused on:
Data Collection
Chatbots are fed with large datasets of conversational and customer data, often comprising text-based interactions between humans. These datasets may be created by businesses or sourced from public conversations on the internet. The more data available, the better the chatbot's learning potential.
Preprocessing
Raw conversational data often needs preprocessing. This involves tasks like tokenization (breaking text into words or phrases), removing stop words (common words like "the" or "and" that don't carry significant meaning), and stemming (reducing words to their root form).
Training Algorithms
Chatbots utilize advanced machine learning algorithms to identify patterns in conversational data. Recurrent neural networks (RNNs) are structured to recognize relationships between words based on their sequence.
Transformer models, distinct from RNNs, like BERT and GPT-3, leverage the attention mechanism to grasp context effectively. They consider the entire context of an input rather than processing word-by-word. Such algorithms empower chatbots to analyze human conversation patterns and respond naturally.
Feedback Loop
Many chatbot systems incorporate a feedback loop mechanism where human operators review and correct chatbot responses. This feedback is then used to retrain the chatbot, improving its performance over time.
Contextual Understanding
Advanced chatbots, especially those built on transformer models, are better able to grasp the contextual meaning of conversations. They can understand the meaning of words and phrases based on the context of the entire dialog, rather than just responding to individual statements. This allows them to provide responses that are relevant to the overall conversation flow and context, rather than just replying to isolated sentences.
Current machine learning enables chatbots to hold natural conversations during live chat interactions, but significant gaps remain in emulating human skills. Despite best efforts to minimize biases, limitations in training data can still lead chatbots astray. While AI assistance shows promise in customer service, frustrated customers often need a connection and compassion that only humans can provide.
Leveraging Chatbots: Balancing Strengths and Limitations
Chatbots offer noteworthy advantages but also face challenges that require balancing their capabilities against human needs.
The Benefits
Chatbots provide instant, 24/7 responses for recurrent customer queries, ensuring consistent and efficient support. By handling repetitive tasks, they free up human agents for intricate problems requiring empathy or nuanced problem-solving. Their data processing capabilities deliver valuable insights to improve experiences.
The Challenges
Chatbots struggle with highly emotional or intricate customer concerns, lacking human discernment. Achieving seamless integration with existing systems poses technical hurdles. Keeping knowledge bases current and refining language processing remain ongoing needs. Data privacy and security risks require vigilance. Careful calibration is necessary to align chatbot interactions with brand tone and values.
Finding the Right Balance
The key is strategically deploying chatbots where they excel while having human agents step in for situations requiring complex communications. Continual monitoring, training and upgrading of chatbot capabilities can further minimize limitations.
For example, a company deployed a chatbot to handle an influx of customer complaints, prioritizing speed and efficiency. However, the chatbot's impersonal responses aggravated emotional concerns, eroding trust and loyalty. This demonstrates the need for human judgment to complement chatbots' efficiency focus.
Relying solely on AI risks worsening customer frustrations and deteriorating satisfaction. The most effective solutions involve human-AI collaboration in customer service, blending chatbot strengths like efficiency and availability with human capabilities like empathy and complex problem-solving. Ongoing improvements to chatbot training and integration are essential to maximize their value while avoiding over-reliance on AI alone.
With the right human-AI balance, businesses can deliver responsive, personalized support across channels to satisfy and retain customers.

The Struggle with Emotion Detection
When customers get angry, it is often due to unresolved issues, unmet expectations, or poor experiences. To properly address the underlying reasons behind customer frustration, AI chatbots employ various techniques to analyze emotions and intent:
Sentiment Analysis
Through natural language processing, AI analyzes customer messages for sentiment, determining if they are positive, negative, or neutral. This involves examining word choice, emojis, sentence structure, and other linguistic elements to gauge the customer's emotional state accurately.
Intent Recognition
AI chatbots classify customer messages into predefined intent categories such as inquiries, complaints, or requests for information. This categorization relies on training data points that includes sample intents, allowing the bot to recognize the underlying purpose of the customer's message.
Named Entity Recognition
Identifying key entities like product or service names, locations, or people in customer messages is crucial for understanding context. By recognizing these entities, AI chatbots can provide more relevant and helpful responses tailored to the customer's query or complaint.

Dialogue State Tracking
In multi-turn conversations, AI chatbots keep track of the customer's progress and context. This enables them to provide coherent and context-aware responses throughout the conversation, improving overall customer loyalty and experience.
Tone Analysis
AI chatbots go beyond words to detect emotion, aggression, or frustration in the customer's tone and word choice. By assessing the emotional undertones, chatbots can respond with empathy and sensitivity when needed.
Feedback Analysis
AI chatbots continually improve their understanding by reviewing past conversational logs and customer satisfaction ratings. Analyzing feedback helps them refine their responses and adapt to customer preferences over time.
Hybrid Approaches
Many AI chatbots utilize hybrid approaches, combining rule-based systems, machine learning classifiers, and deep learning neural networks. This versatile combination allows chatbots to adapt to various conversation types and complexities, enhancing their ability to understand customers' intentions and emotions accurately.
However, without core human skills like empathy, active listening, and reading between the lines, AI still falls short in navigating charged interactions and forging true understanding. This "empathy gap" persists despite innovations like hybrid deep learning models. Businesses deploying chatbots must augment them with human insight when managing angry customers.
Escalations and the Role of Human Agents
Developing an effective handoff strategy from chatbot to customer service team is key to maintaining this balance and upholding a customer-centric service philosophy.
By designating specific scenarios for handoffs, such as multiple failed responses, high urgency tickets, or negative sentiment detection, businesses can ensure chatbots seamlessly transition complex conversations to support agents.
It's thereafter that human customer service agents, with proper training in empathy, active listening, and conflict resolution, can provide the understanding needed to resolve charged interactions, alleviate customer frustrations, and rebuild loyalty. This human touch aligns with a customer service philosophy focused on understanding and satisfying consumer needs.
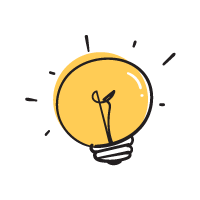
Tip: Over-dependence on automation can compromise customer satisfaction. The key is blending AI's capabilities with human emotional intelligence, ensuring responsive, compassionate support across all touchpoints.
Neglecting these human-centered capabilities and over-relying on automation risks damaging customer satisfaction, underscoring why handoffs remain critical. Combining AI efficiency with human emotional intelligence, businesses can deliver responsive, personalized support across all channels and scenarios, in line with a philosophy grounded in compassion.
The ultimate goal is creating a unified customer experience where chatbots and agents complement each other according to their strengths. With the right strategy, businesses can maintain the optimal balance while staying true to customer-focused values.
Managing Expectations Towards More Empathetic AI
Integrating AI and human expertise emerges as a powerful combination. Each brings unique strengths to the table, creating a symbiotic relationship that holds immense promise for delivering exceptional customer service.
AI's proficiency in handling high volumes of routine queries efficiently is undeniable, providing customers with quick responses and streamlined interactions. However, as our interactions become increasingly complex and emotionally nuanced, the indispensable human touch comes into play, offering empathy and adeptness in addressing multifaceted concerns.
Customer expectations have evolved, with a growing demand for personalized and empathetic service experiences. While AI alone may not yet replicate human-level empathy in customer service, it can significantly enhance human efforts by automating routine tasks, providing data-driven insights, and facilitating faster issue resolution.
The future of customer service lies in the collaboration between AI and support agents, where technology augments the capabilities of human operators, ensuring that each customer interaction remains personal, meaningful, and tailored to their unique needs.
Enhance your customer service by integrating AI and human expertise. Contact us today to discover how our solutions can improve your customer interactions, combining efficiency and empathy for exceptional service experiences.