Effective knowledge management is the foundation of success for businesses today. A well-organized knowledge base empowers employees with the information they need to solve complex problems, deliver exceptional customer support, and ultimately drive customer satisfaction.
Imagine a scenario where a website visitor needs urgent assistance. They have a specific question or problem, and they are looking for a quick solution. Without a knowledge base, they would have to navigate through numerous pages, contact the support team, or scan through irrelevant content, leading to a frustrating experience.
However, with a well-organized and easily accessible knowledge base, the visitor can simply enter their query into the search bar or browse through the categorized articles.
Yet, the challenge lies in maintaining and harnessing the large amount of information stored within these knowledge bases. That's where AI-powered search comes into play, ready to revolutionize and enhance knowledge management practices across industries.
Unlike a traditional search engine which relies on keyword indexing, AI powered search is powered by machine learning (ML), natural language processing (NLP), and artificial intelligence (AI) including semantic vector search, intelligent search, and cognitive search. This AI-powered search is a game-changer that understands user intent and delivers precise results instantly, simplifying knowledge retrieval.
In this blog, we will explore how AI-powered search transforms knowledge bases management and efficiency.
The Role of Natural Language Processing in Search
What is NLP?
Natural language search, also known as “conversational search,” uses natural language processing to allow computers to understand and interpret human language. NLP enables computers to detect patterns and relationships between words to grasp people's true intent.
NLP search engines are designed to comprehend slang, filler words, typos, synonyms, multiple languages, and frequently asked questions.
How does NLP work?
Natural language processing (NLP) is a field that combines computational linguistics, machine learning, and deep learning techniques to process human language. Computational linguistics involves the development of advanced algorithms and models that can understand, represent, and manipulate natural language, and has applications in many important areas such as language translation, information retrieval, and speech recognition.
Semantic analysis is a method used to examine the meaning of a text. Unlike syntactic analysis, which focuses on analyzing the grammatical structure of a sentence, the goal of semantic analysis is to understand the purpose of the text. These methods are employed to develop frameworks that enable machines to comprehend conversational language.
Machine learning, on the other hand, trains computers to improve their efficiency by using sample data. NLP applications rely on machine learning methods to recognize complex language features like sarcasm, metaphors, variations in sentence structure, and grammar exceptions.
Deep learning, a subset of machine learning, teaches computers to think and learn similarly to humans.
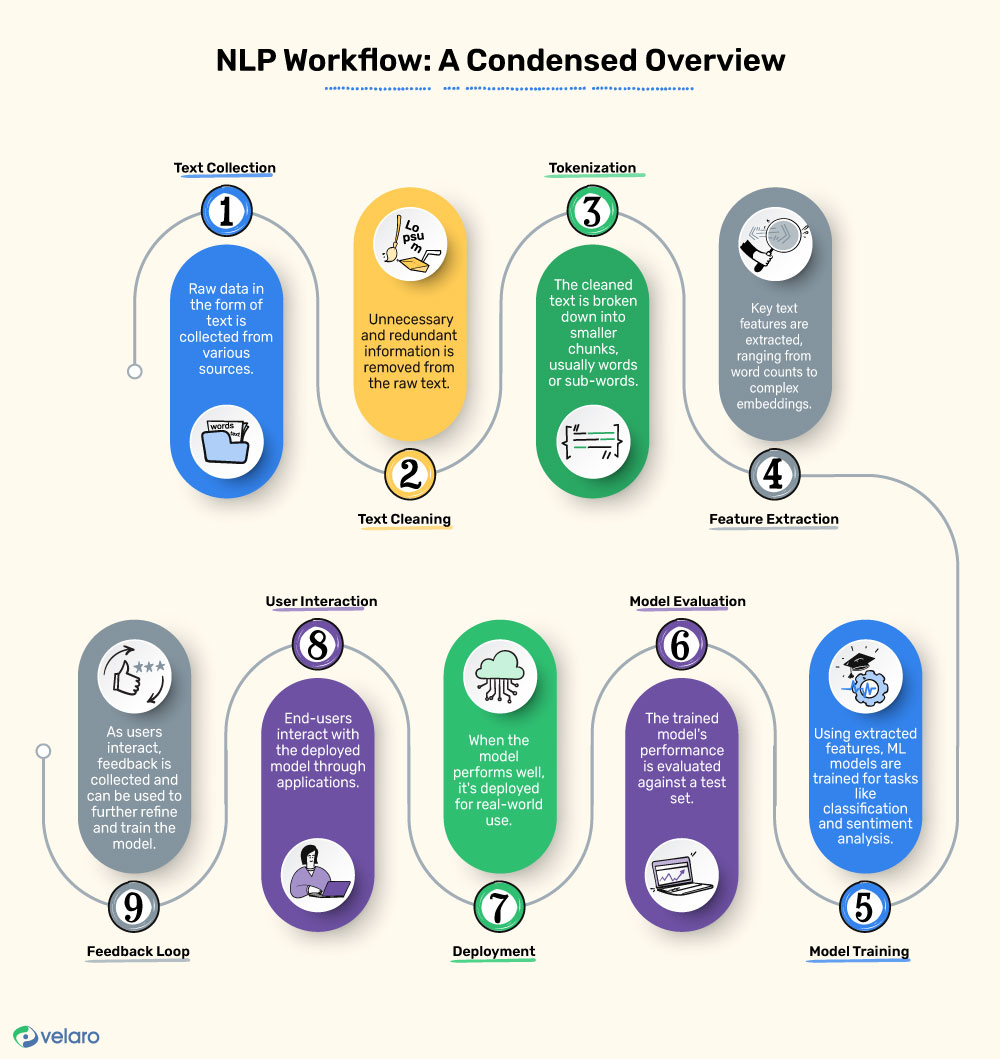
The process of implementing NLP typically involves gathering and preparing unstructured text or speech data from various sources such as cloud data warehouses, surveys, emails, or business applications. Pre-processing techniques like tokenization, stemming, lemmatization, and stop word removal are then used to refine the data for specific applications.
Tokenization breaks sentences into individual words or phrases, while stemming and lemmatization simplify words into their root form. Stop word removal eliminates insignificant words that do not contribute to sentence meaning.
Researchers feed the pre-processed data into NLP models, combining it with machine learning algorithms to train the models on specific applications. After training, the NLP models are deployed or integrated into existing production environments.
How NLP has Transformed Traditional Keyword-based Searches
Natural Language Processing (NLP) has changed how users perform online searches. In the past, keyword-based searches led to irrelevant or missed results. NLP has changed this by interpreting phrases in natural language, understanding context, and detecting user intent. An NLP engine can recognize the intent of a query's structure, overcome regional language differences and even account for slang and typos providing a more tailored and accurate search result.
NLP is a disruptive technology that is constantly evolving. By relying heavily on training data, it can still struggle with more complex or ambiguous queries. It is crucial to continually refine NLP models to ensure fairness and accuracy in search results.
Even though NLP has been in the picture for some time, the recent surge in deep learning techniques combined with heightened computational power has catapulted it to the forefront of the search experience. This prominence is underscored by breakthroughs like GPT-3 and other advanced language models.
Benefits of AI-Powered Search for Knowledge Bases
AI-powered knowledge base systems offer a range of benefits that significantly improve the user experience and provide efficient access to information. These benefits include:
Enhanced Search Accuracy
One of the key advantages of AI-powered search for knowledge bases is its ability to deliver highly accurate search results. Traditional keyword-based searches often generate a large number of irrelevant results. AI-powered search systems use advanced algorithms to understand user intent and context, allowing them to provide highly relevant results based on the actual meaning of the query.
Personalized Recommendations
Another benefit of AI-powered search is the ability to provide personalized recommendations. By analyzing user behavior, preferences, and historical data, the search system can suggest related articles, relevant documents, or even predict the next action based on the user's past interactions that results in increasing engagement and productivity.
Leveraged NLP Capabilities for User-Friendly Search
AI-powered search systems can also leverage NLP capabilities to understand and process natural language queries. This means users can interact with the search system using everyday language, rather than being limited to specific keywords or phrases. NLP enables the system to understand context, synonyms, and variations in language, ultimately improving the accuracy and effectiveness of search results.
Improved Productivity
AI-powered search systems improve productivity through intelligent features such as auto-complete, spell-check, and query recommendations. Auto-complete provides real-time query suggestions as users type, accelerating information retrieval. Spell-check automatically corrects misspellings and reduces errors. Query recommendations suggest relevant options based on the user's query, enabling more precise and accurate results.
Real-world Examples of AI in Knowledge Base Search
Artificial intelligence contributions to enhancing knowledge base search have been immense, particularly in industries that rely heavily on quick and precise information retrieval. Let’s dive into a few industry-specific instances:
1. Sales Team Product Knowledge
In sales, time is often of the essence. AI-powered search within a company’s product knowledge base can instantly surface product or service details, comparisons, and benefits, helping sales reps to answer client questions on the fly and tailor pitches effectively.
2. Marketing Content Libraries
Marketers often maintain libraries of content ranging from blogs to whitepapers. AI-enhanced search can help them pinpoint specific content pieces, track their performance, and even suggest gaps in content strategy based on search trends.
3. Customer Service Troubleshooting
When customers encounter problems, service reps need to find solutions quickly. Utilizing both an external knowledge base for public documentation and an internal knowledge base for proprietary solutions, an AI-augmented system can interpret customer descriptions, no matter how vague. This helps direct reps to the most relevant troubleshooting steps or documentation customized to the issue at hand.
4. Product Recommendations in E-commerce
E-commerce platforms maintain large knowledge bases of products. AI can interpret a customer’s search patterns, preferences, and past purchase history to recommend products that are most likely to interest them.
5. Training and Onboarding
For industries with frequent staff turnover or complex processes, AI-powered search within training databases can help new employees find necessary resources, understand company protocols, and ramp up more swiftly.
6. Voice Search
Voice recognition integration with AI-powered search allows for hands-free access to knowledge base content, which is especially useful when typing or navigating is not possible for users.
7. Visual Search
In addition to text-based searches, AI systems can support visual search capabilities. Users can upload images or screenshots of products or issues they need assistance with, and the system can analyze and provide relevant information or answers based on the visual content.
8. Trend Analysis
Customer search behavior and patterns can be analyzed through an AI which helps identify emerging trends or topics of interest. This information can be used to create new content and update existing articles, ensuring that the most relevant and up-to-date information is available to customers.
9. Advanced Filter and Sorting Options
Customers can refine their search results based on various criteria, such as relevance, date, popularity, or specific attributes, to quickly find the information or solutions they need. AI improves the filtering and sorting capabilities of a knowledge base tool.
10. Real-time Updates and Notifications
AI-powered search systems can enable real-time updates and notifications for users. This includes informing customers about the availability of new articles, updates to existing content, or alerts related to their specific areas of interest or subscribed topics.
The Future of Knowledge Base Search
The future of knowledge base search holds immense potential for advancements and innovation. With the continuous evolution of technology, we can expect smarter search tools to emerge, catering to the increasing demands of the users. One notable prediction for the future is the integration of natural language processing (NLP) capabilities into knowledge base search engines. This would allow users to ask complex questions and receive precise and contextually relevant answers.
Moreover, NLP can optimize the personalization of search results by tailoring them to individual preferences and needs. As machine learning algorithms gain more prevalence, knowledge base search engines will become better at predicting users' search intent, providing more accurate and relevant results. These advancements enable knowledge base search engines to provide superior and more efficient customer service, leading to higher satisfaction rates and brand loyalty.
Take your knowledge base solution to the next level. Connect with our team to learn more about Velaro's knowledge base software.